Computers can map your route to work, automatically turn speech into written text - and predict very early stages of dementia with more than 80 per cent accuracy.
Language Sciences members Giuseppe Carenini, professor of Computer Science, Hyeju Jang, Computer Science postdoctoral fellow, and Neurology professor Thalia Field worked with Computer Sciences graduate Weirui Kong to develop accurate machine learning algorithms that can predict dementia based on language patterns.
There are no effective therapies to modify the course of Alzheimer’s disease, Field said, and current thinking is that a successful disease-modifying drug will work in people with very early stage disease.
“So we’re trying to figure out a non-invasive and quick way to identify people that might be good candidates for future trials of dementia treatments.”
If computers can help predict dementia based simply on speech, this would also provide a low cost way of identifying patients early on, a boon to countries with limited resources, Carenini said. Even countries like Canada would benefit, Field said, with machine learning models removing the need for invasive tests like spinal taps, or accessibility barriers such as waitlists, and the cost of advanced scans.
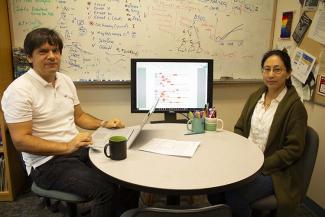
Professor Carenini and Dr. Jang explain their work on the paper 'A Neural Model for Predicting Dementia from Language.'
The team’s research makes use of a dataset from the 1980s comprising audio recordings and transcriptions of 257 dementia patients and 242 healthy elderly controls, who described the scene in a picture, ‘The Cookie Theft’. Using 90% of this data, Carenini, Jang, and Kong trained the model, and then tested it on the remaining 10% to see if it could accurately predict dementia patients.
In a paper presented at the Machine Learning for Healthcare conference last month, on which Kong was first author, they describe how using a neural network, a relatively new form of machine learning algorithm, and inputting age as a feature into the model, it achieved 86.9% accuracy in predicting patients with dementia, extending previous work of 84.4%.
Using the neural network, the model was also picture-agnostic. Previous models used information units defined by human clinicians, or objects and actions appearing in the picture (for the ‘Cookie Theft’ image, these were words such as mother, stool, and overflowing.) But these were specific to the particular picture, and would need to be re-defined when a different picture, or language, were used. A model that did not rely on inputted information units could be generalized to other cultures and languages with the appropriate training data, the researchers concluded.
The model captures language patterns as it works, Jang said, which researchers can analyze to see whether there are any interesting results or contributions to dementia research. “We can capture those language patterns as well as predict new things.” One pattern they found was that their model tended to select information units as important in predicting whether someone had dementia or not – the same words human experts had also identified – but did not attend to all information units uniformly.
Currently, Jang, Carenini, and Field, with Computer Science professor Cristina Conati, are gathering new data, including eye tracking, based on studies which suggest people may pay attention to words differently if they have early signs of dementia. Machine learning in healthcare is a very collaborative area of research, Carenini said.
“At all levels you need clinicians, you need computer scientists, and then you need more and more people that are trained in both fields and can understand their interface.”
Main image by Gerd Altmann from Pixabay