Recent progress in artificial intelligence (AI) is transforming lives. In particular, advances in deep learning, a subfield of AI that loosely mimics information processing in the brain, has enabled innovations across a wide range of domains. One area where rapid progress is taking place is machine translation.
In their recent work, ‘TURJUMAN: A Public Toolkit for Neural Arabic Machine Translation’, Prof. Muhammad Abdul-Mageed and two Postdoctoral fellows in the Deep Learning and NLP Group, Dr. El Moatez Billah Nagoudi and Dr. Abdelrahim Elmadany, took on this machine translation challenge. The team packaged the model into a tool called ‘Turjuman,’ which is Arabic for ‘translator’ or ‘interpreter’, and have released it for public use.
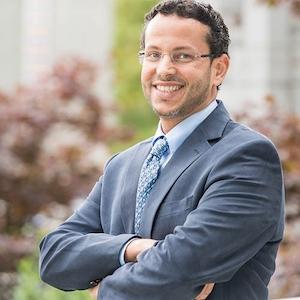
Machine translation is the task of automatically translating from one language to another, and is particularly challenging due to ambiguity inherent in language. Even human translators make mistakes and disagree on how to translate a piece of text. This is because each language has its own grammar and way of arranging elements such as words and phrases to form sentences and paragraphs. People from different places, age groups, and social classes all use language differently. Even the same person may use language differently based on who they are talking to; whether they are happy, surprised, or sad (i.e., their emotion). Translating all these nuances of meaning from one language into another, across different cultures, is by no means an easy task.
“In spite of the exciting progress we have made, translation itself remains an intrinsically complex task,” said Abdul-Mageed. “Think about data availability. While we are able to create good systems when we have sufficiently large parallel data from a given language pair, it is still hard to develop models with smaller sized datasets. For the majority of the world’s 7000+ known languages today, we have reasonably large data from only around 100 languages.”
The team developed a single deep learning model that translates from 20 different languages into Arabic. Supported languages include those from which there are sizeable amounts of data such as English, French and Spanish, as well as those with less data like Cebuano, Tamashek, and Yoruba. The team focused on translating into Arabic since Arabic has rich morphology, which makes generating Arabic text challenging, as words carry significant grammatical information such as number and gender.
Dr. Abdul-Mageed shared that one of the biggest challenges the team faced was with computing infrastructure, so they collaborated with Advanced Micro Devices (AMD) to train the translation models at the core of Turjuman.
“Current deep learning models are practically trainable only on graphical processing units (GPUs), since these are most suited to heavy mathematical operations such as matrix multiplication typical of these systems,” said Abdul-Mageed. “Machines with sufficient GPUs are expensive. While we at UBC have done a great job so far in making available GPU servers such as Sockeye, the needs of my group are currently so high that Sockeye and other national systems alone are not enough.”
Turjuman exploits the AraT5 model, a sequence-to-sequence Transformer-based model developed previously by Prof. Abdul-Mageed’s Deep Learning and NLP Group. AraT5 endows Turjuman with a powerful ability to decode into Arabic. The toolkit offers the possibility of employing a number of diverse decoding methods, making it suited for acquiring paraphrases for the Arabic translations as an added value.
“Our hope is that [Turjuman] will help accelerate research in this area by serving as a reasonable baseline to compare to. We also hope it will have applications in education, such as language learning, health, recreation and more,” said Dr. Abdul-Mageed. “In my experience, once the technology is created, people will find many ways to make use of it. We hope this will be the case for Turjuman.”
The paper was published in ‘The 5th Workshop on Open-Source Arabic Corpora and Processing Tools’ (OSACT5) at the ‘14th Conference on Language Resources and Evaluation’ (LREC 2022) where it received the Best Paper Award.
Prof. Abdul-Mageed and the team have provided detailed information about how to download and use Turjuman at the group GitHub page. For additional information, view Prof. Abdul-Magee’s Twitter thread about Turjuman, and click here to try a demo based on the software.
Written by Kelsea Franzke